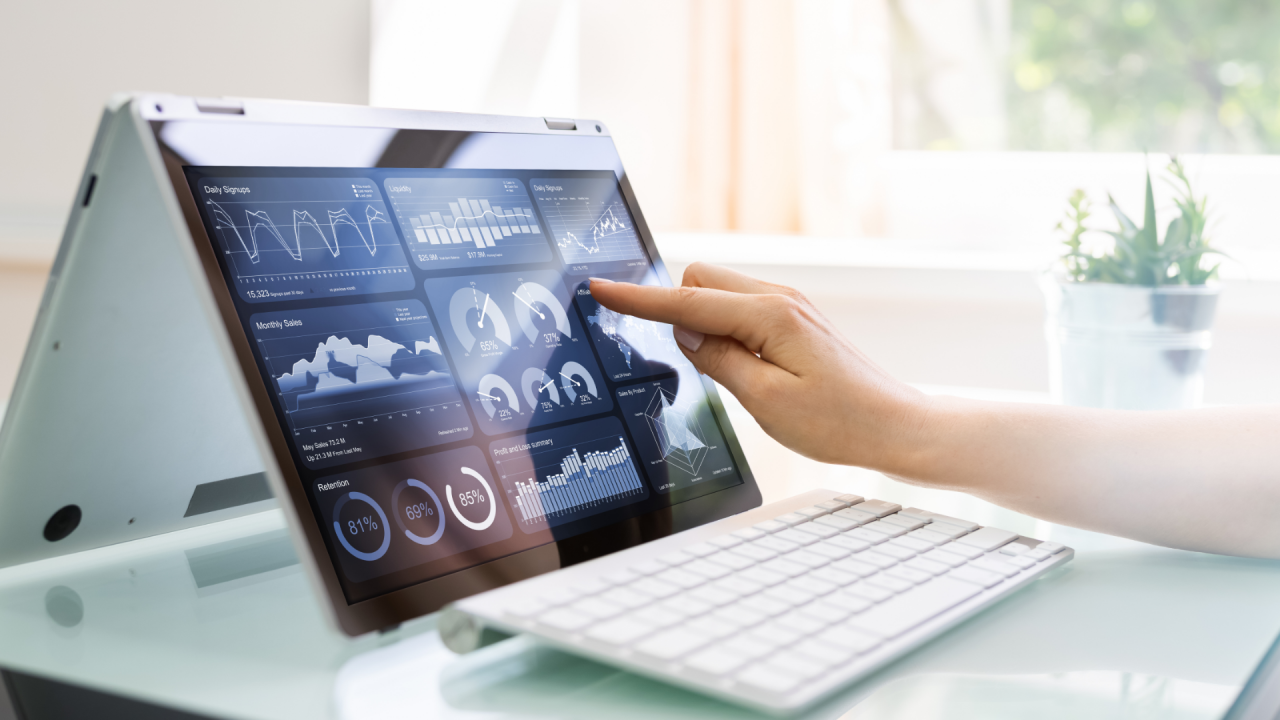
Data Thinking for startups
Data Thinking is a methodology for designing use cases for data analytics solutions in interdisciplinary teams, heavily inspired by the design thinking methodology and a startup mindset. It is a way to communicate about data and to make data science more approachable and fun for everyone. If you aim to develop data-driven products, Data Thinking will be a must-have for you. But beforehand, let’s clarify why you need data-driven products in the first place.
No Digitalization without Data
There has been a massive market for digital transformation, disruptions, and various new digital business models for all kinds of organizations for many years. Digitalization is the heart of the startup ecosystem, as startups are modern, fast, agile, and lightweight enough to rethink processes and start from scratch with all the new technological possibilities.
Let’s take a step back and think about that famous buzzword. The word ‘digitalization’ itself originates from the Latin digitus, meaning ‘finger’ and came to be by showing a finger as 1 or no finger as 0. The transformation would be the conversion of analog information into digital signals, i. e. discrete values. One trivial example constitutes scanning a piece of paper and recognizing the patterns of ink on the paper to compose layout, letters and sentences. This text could be interpreted by all kinds of different software to make it searchable, or analyzed regarding its use of words, readability, semantic content and even emotions. Once the information on the paper turns into data that can be processed by software, all kinds of new use cases are possible.
There are two common major views of digitalization. The more mainstream approach is to optimize processes by using computers for automation where no computers have been used before. But it is a more relevant interpretation that the variety of life is being transformed into measurable and standardized values or codes. Think of it as determining the date and time. Before we had clocks, we could only approximate the time and had to agree on a standard way of communicating it. Combine that with everything exposing interfaces for sharing, mixing and matching data to make all relevant information available to everyone who needs it.
Three things are apparent:
1. Digitalization without data is not possible. Where use cases are, there is data.
2. Proper use cases are required to select the required data. Use cases need to be designed by people with proper domain knowledge in collaboration with data scientists.
3. A general understanding of data analytics methodology, usage and ethical understanding a.k.a. data literacy, is much needed in organization’s leadership and all departments to identify use cases for digitalization.
Meaningful Use cases
In a production facility, historical and current values are being measured to provide information about the state of a machine. With this maintenance, measurements are taken when they are required and the lifetime of used parts is being extended, saving costs.
Using pattern recognition, all sorts of anomalies can be detected, hinting at problems before they occur. Processes can be checked and optimized for profitability and quality for optimization using statistics and machine learning if the right data is available.
There is a vast number of use cases for optimizing processes in organizations or coming up with new business models, utilizing data analytics. A return on investment for the required technology, implementation and building up the knowledge can be reached quickly, if a suitable approach is selected. Startups know the proper way of maximizing output with minimal investment: figuring out the right use cases for implementation by validating assumptions, prototyping rapidly, failing fast and iterating by learning from past successes and also failures.
The struggle of the data-driven company
Multiple, partly conflicting studies suggest several criteria for organizations’ progress on the track of becoming a data-driven company. In short, a data-driven company is characterized by the following aspects:
1. Data is a core element for daily work
2. Real insights are derived from good quality data
3. Facts (data) are the basis for change and not gut feeling
A recently published peer-reviewed study by Giudice da Silva Cezar and Maçada (2021)[1] confirms that data literacy as ‘a wide knowledge base that enables professionals to transform data into information’ is positively associated with individual performance on the one hand and has a negative association relating to perceived data overload on the other hand. The latter, in turn, reduces individual performance while correlating with technostress, a state of extremely negative affection with a crucial impact on employees’ functioning. In other words, gaining skills regarding working with data can be seen as a central factor in both performance and satisfaction improvement of employees. This perspective can be further replenished by a study completed by Härtwig and Sapronova (2021) [2], whose model suggests, among other points, an impact of self-efficacy on job commitment specifically in a digitalization context.
When we talk about data-driven innovation and digitalization, we actually face a cultural challenge of empowering all people with the necessary skills, toolset and ethics to successfully work with data.
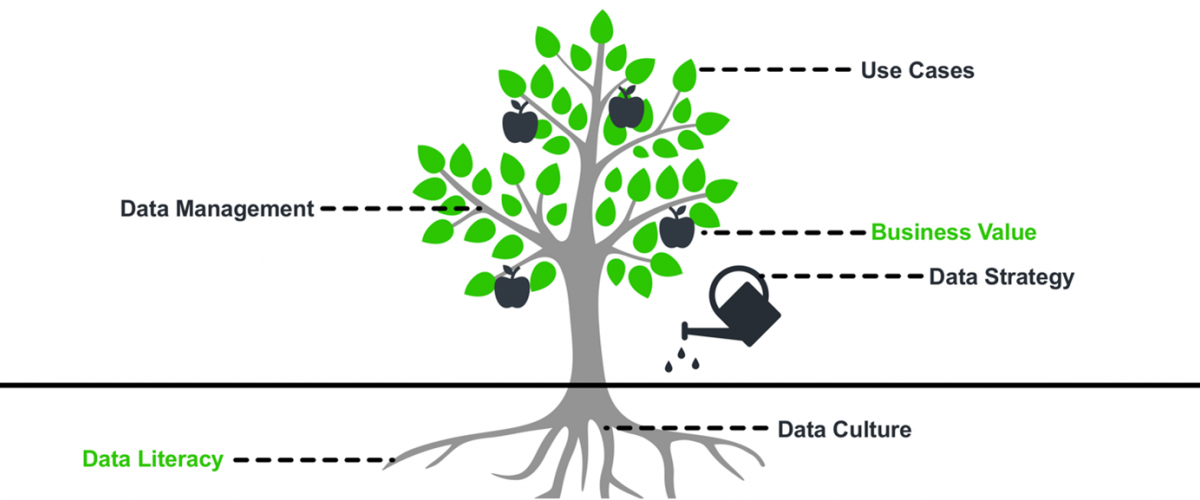
This is a huge advantage for startup companies as they usually start fresh. They start with little restraint from trying new technology and methods, but, on the other hand, also with little data.
The following pain points are usually signs of a lack of data culture in companies:
- Data and reports/analyses are kept in silos
- There is an uncontrollable data overload
- Interpretation of data is too hard
- Cleaning of raw data is hard
- Hardware limitations do not allow processing and calculation of the amount of data
- Security and GDPR are hard to evaluate threats to working with data
- Technology and skills are lacking
What is Data Thinking?
Data Thinking is a methodology for identifying and prototyping first drafts of useful and measurable use cases, addressing the pain points above. By quickly building functional proof of ideas the data culture will be fostered. Using Data Thinking, countless methods and technologies of data science will be made tangible and understandable to all people, who are not data specialists. In a collaborative fashion the skills of data scientists, as well as the domain knowledge of business departments will be used to identify use cases that yield value to the organization. These skills are real assets, for example through delivering measurable profit.
In the Data Thinking workshop format, proven methods of Design Thinking are being combined with Data Science techniques. Through Design Thinking, actual needs and real drafts can be identified. In addition to that quick and cheap minimum viable products (MVP) can be implemented. The data scientists attending the workshop will ensure functioning prototypes and measurability of success or failure.
Using phases and methods from the Design Thinking model, business use cases can be constructed in a creative way. From the outset, the focus is being put only on such use cases which can be improved or realized using data. The most important factor is the business impact of those use cases. By analyzing customer needs and their pains and gains in a value case canvas, use cases are being found and validated if they really meet a need. Then users or customers provide actual feedback. This ensures early elimination of use cases that have no value for the target group, thus guaranteeing customer-centricity of the approach.
Important questions of Data Thinking concern the spot of ‘separating the wheat from the chaff’ or fields where people would like to have a look into the future. In case these types of questions are interesting for a business, Data Science can be particularly helpful. Provided that the necessary data can be identified.
Data Science Methods
After accomplishing the first phases of Design Thinking, we reach the ideation phase, where the main part of the Data Thinking Workshop takes place, namely The Data Thinking Canvas.
The Data Thinking Canvas is derived from the Business Case Canvas, well known in the startup ecosystem. It adds central requirements for data science solutions needed for the following steps of the workshop and implementation. While conceptualizing the use cases, attendees will need to permanently contemplate sources of primary data, select secondary data to be generated and consider data that might be present somewhere in the indiscernible background, not even explicitly known to the company yet.
An example for the latter: many companies employ field service workers, who could generate movement profiles using their smartphones. After removing personally identifiable information, hence complying to possible privacy policies, new insights about several processes can be gained.
Selecting relevant data early in the process can help filter the overload of useless records. On the other hand, addition of external data is being considered as well. A value can be created, for example, by buying data off the market, or by using freely available public data. Sociodemographic data or various surveys can also vitally contribute to gaining insights.
When use cases are prioritized by profitability and expectation of success, other factors also play a role, such as complexity, effort of data acquisition and the perceived practical benefit for the users, or even new data’s potential for future analyses.
Quick, functioning Prototypes
When a use case was selected, a testable, functioning prototype should be developed as soon as possible. This phase is carried out by the data scientists. With a minimal effort they try to implement a first working prototype, which is not yet fully automated and might not provide the final architecture. But it is useable for validating impact hypotheses as well as going through the user experience. The data scientists chose appropriate methods and technologies to translate to the desired result.
If the prototype manages to deliver the expected results it can be built as a sustainable and clean production solution. Successful implementations demonstrate the meaning of data in the organization and foster rethinking or reevaluating work with data. The more use cases are being realized this way, the more data will become relevant for future solutions. People will think more in data terms and the maturity on the way towards a data-driven company will grow.
Author: Manuel Achterberg, Management Consultant at Cassini Consulting AG
Cassini Consulting AG
Cassini Consulting AG is a leading consulting firm in the field of digitalization. Cassini focuses on topics such as digital transformation, business building, digital marketing, IT strategy, innovation processes and much more. Many of these areas are highly relevant for our B2B startups. Our startups can tap into the broad knowledge pool of Cassini while Cassini experts get immediate access to new technologies.